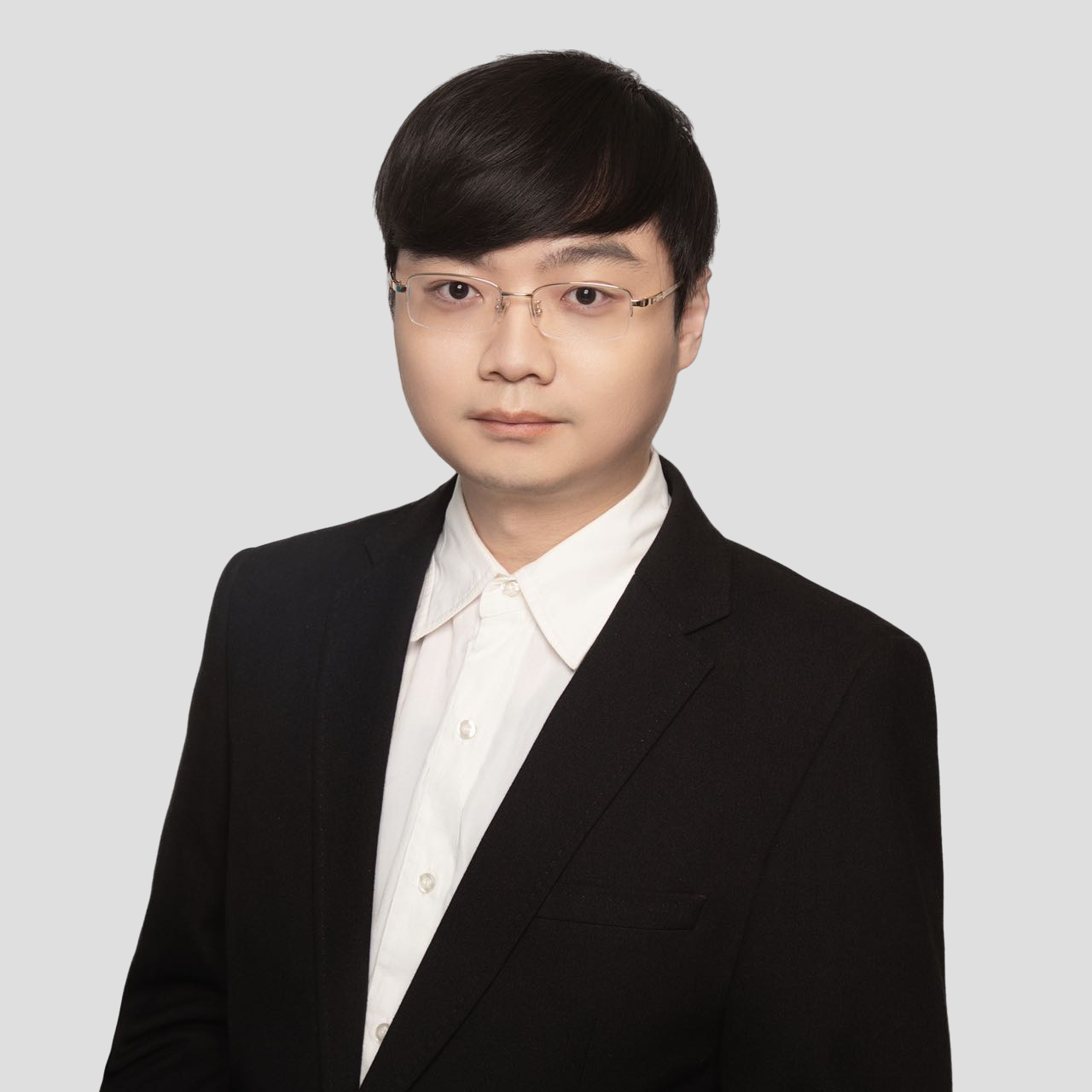
Li Yang
PhD
Assistant Professor
Networking and IT SecurityFaculty of Business and Information Technology
Dr. Li Yang's research focuses on applying AI and machine learning to cybersecurity, with a particular emphasis on intrusion detection and anomaly detection in 5G/6G networks and IoT systems. This involves the development of optimized and Automated ML (AutoML), concept drift adaptation, online learning, and continual learning techniques to enhance cybersecurity measures. Additionally, his work extends to the realms of trustworthy AI and AI security, specifically addressing adversarial machine learning attacks and defense strategies.
Languages
English, Chinese
- Graduate student research supervision
- Media inquiries
- Partnerships
li.yang@ontariotechu.ca
905.721.8668 ext. 3806
- PhD - Electrical and Computer Engineering Western University, London 2022
- MSC - Engineering University of Guelph 2018
“On AutoML for Intrusion Detection in 6G and IoT Systems: From Theory to Practice”, A Tutorial in 2024 IEEE World Forum on Public Safety Technology (WF-PST)
Washington, DC, USA May 1, 2024Journal Papers
- L. Yang, M. El Rajab, A. Shami, and S. Muhaidat, “Enabling AutoML for Zero-Touch Network Security: Use-Case Driven Analysis,” IEEE Transactions on Network and Service Management (Impact Factor: 5.3), pp. 1-28, Mar. 2024.
- El Rajab, L. Yang, and A. Shami “Zero-Touch Networks: Towards Next-Generation Network Automation,” Computer Networks (Impact Factor: 5.6), Elsevier, vol. 243, p. 110294, Feb. 2024.
- L. Yang and A. Shami, "A Multi-Stage Automated Online Network Data Stream Analytics Framework for IIoT Systems," IEEE Transactions on Industrial Informatics (Impact Factor: 11.648), vol. 19, no. 2, pp. 2107-2116, 2023.
- L. Yang and A. Shami, “IDS-ML: An open source code for Intrusion Detection System development using Machine Learning,” Software Impacts (CiteScore: 1), Elsevier, vol. 14, p. 100446, 2022.
- L. Yang and A. Shami, “IoT Data Analytics in Dynamic Environments: From An Automated Machine Learning Perspective,” Engineering Applications of Artificial Intelligence (Impact Factor: 7.802), Elsevier, vol. 116, pp. 1–33, 2022.
- L. Yang, A. Moubayed, A. Shami, P. Heidari, A. Boukhtouta, A. Larabi, R. Brunner, S. Preda, and D. Migault, “Multi-Perspective Content Delivery Networks Security Framework Using Optimized Unsupervised Anomaly Detection,” IEEE Transactions on Network and Service Management (Impact Factor: 4.758), vol. 19, no. 1, pp. 686-705, Mar. 2022.
- L. Yang, A. Moubayed and A. Shami, “MTH-IDS: A Multitiered Hybrid Intrusion Detection System for Internet of Vehicles,” IEEE Internet of Things Journal (Impact Factor: 10.238), vol. 9, no. 1, pp. 616-632, Jan. 2022.
- L. Yang and A. Shami, “A Lightweight Concept Drift Detection and Adaptation Framework for IoT Data Streams,” IEEE Internet of Things Magazine (SJR: 2.607), vol. 4, no. 2, pp. 96-101, Jun. 2021.
- L. Yang and A. Shami, “On Hyperparameter Optimization of Machine Learning Algorithms: Theory and Practice,” Neurocomputing (Impact Factor: 5.779), Elsevier, vol. 415, pp. 295–316, 2020.
- L. Yang, R. Muresan, A. Al-Dweik and L. Hadjileontiadis, "Image-Based Visibility Estimation Algorithm for Intelligent Transportation Systems," IEEE Access (Impact Factor: 3.476), vol. 6, pp. 76728-76740, 2018.
Book Chapters
- L. Yang, A. Moubayed, A. Shami, A. Boukhtouta, P. Heidari, S. Preda, R. Brunner, D. Migault, and A. Larabi, “Forensic Data Analytics for Anomaly Detection in Evolving Networks”, A book chapter in Innovations in Digital Forensics, World Scientific, 2023.
Conference Papers
- L. Yang and A. Shami, “A Transfer Learning and Optimized CNN Based Intrusion Detection System for Internet of Vehicles,” in 2022 IEEE International Conference on Communications (ICC), 2022, pp. 1-6.
- L. Yang, D. M. Manias, and A. Shami, “PWPAE: An Ensemble Framework for Concept Drift Adaptation in IoT Data Streams,” in 2021 IEEE Global Communications Conference (GLOBECOM), 2021, pp. 1-6.
- D. M. Manias, I. Shaer, L. Yang, and A. Shami, "Concept Drift Detection in Federated Networked Systems," in 2021 IEEE Global Communications Conference (GLOBECOM), 2021, pp. 1-6.
- L. Yang, A. Moubayed, I. Hamieh, and A. Shami, “Tree-based Intelligent Intrusion Detection System in Internet of Vehicles,” in 2019 IEEE Global Communications Conference (GLOBECOM), 2019, pp. 1-6.
- World's Top 2 per cent Scientists, Stanford/Elsevier, 2024
- Best Paper Award, The Workshop on AutonomousCyber 2024, ACM CCS 2024
- Outstanding Volunteer Award, IEEE London Section, 2023
- Graduate Student Award for Excellence in Research, London, Canada, 2022
- Graduate Symposium Award for Best Presentation, London, Canada, 2022
- Mitacs Accelerate Fellowship, 2021
- The OC2 Lab Industrial Research Excellence Award, London, Canada, 2020
- The OC2 Lab Research Excellence Award, London, Canada, 2020
Western University, London
- Machine Learning
- Attack and Defense
- Special Topics in Information Technology