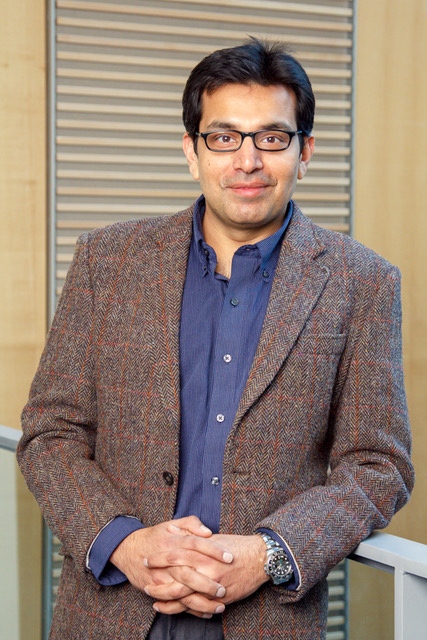
Faisal Qureshi
PhD
Professor
Graduate Program Director
Computer ScienceFaculty of Science
Dr. Qureshi's research focuses on computer vision, and his scientific and engineering interests center on the study of computational models of visual perception to support autonomous, purposeful behavior in the context of camera networks and self-organizing visual sensor networks.
- PhD - Computer Science University of Toronto, Toronto, Ontario 2007
- MSc - Computer Science University of Toronto, Toronto, Ontario 2000
- MSc - Electronics Quaid-e-Azam University, Pakistan 1995
- BSc - Mathematics & Physics (Minor) Punjab University, Pakistan 1992
A Residual-Dyad Encoder Discriminator Network for Remote Sensing Image Matching
Published in IEEE Transactions on Geoscience and Remote Sensing p.14N. Khurshid, Mohbat, M. Taj, and F. Qureshi
2019
Joint Spatial and Layer Attention for Convolutional Networks
Published in Proc. 30th British Machine Vision Conference (BMVC19) p. 14T. Joseph, K. Derpanis, and F. Qureshi
September 2019
Neural Networks Trained to Solve Differential Equations Learn General Representations
Published in Proc. The Thirty-second Annual Conference on Neural Information Processing Systems (NuerIPS 18) p. 11M. Magill, F. Qureshi, and H. de Haan
December 2018
Fast Estimation of Large Displacement Optical Flow Using Dominant Motion Patterns & Sub-Volume PatchMatch Filtering
Published in Proc. 14th Conference on Computer and Robot Vision (CRV 17) p. 8M. Helala and F. Qureshi
Best Computer Vision Paper (May 2017)
Stereo Reconstruction of Droplet Flight Trajectories
Published in IEEE Transactions on Pattern Analysis and Machine Intelligence Volume: 27, Issue: 4 April 1, 2015Luis A. Zarrabeitia, Faisal Z. Qureshi & Dhavide A. Aruliah
This article presents the development of a new method for extracting 3D flight trajectories of droplets using high-speed stereo capture. Results suggest that, even when full stereo information is available, unsynchronized reconstruction using the global motion model can significantly improve the 3D estimation accuracy.
View more - Stereo Reconstruction of Droplet Flight Trajectories
Smart Camera Networks in Virtual Reality
Published in IEEE volume 96, issue 10, pp. 1640-1656F. Z. Qureshi and D. Terzopoulos
Special Issues on "Smart Cameras" (October 2008)
Intelligent Perception and Control for Space Robotics: Autonomous Satellite Rendezvous and Docking
Published in Journal of Machine Vision Applications volume 19, issue 3, pp. 141-161F. Z. Qureshi and D. Terzopoulos
February 2008
IEEE Journal on Emerging and Selected Topics in Circuits and Systems
Published in This research presents a distributed virtual vision simulator capable of simulating large-scale camera networks, and pedestrian traffic in different 3D environments. Specifically, this research shows that the proposed virtual vision simulator can model a camera network, comprising more than one hundred active pan/tilt/zoom and passive wide field-of-view cameras, deployed in an upper floor of an office tower in downtown Toronto.Volume: 3, Issue: 2
This research presents a distributed virtual vision simulator capable of simulating large-scale camera networks, and pedestrian traffic in different 3D environments. Specifically, this research shows that the proposed virtual vision simulator can model a camera network, comprising more than one hundred active pan/tilt/zoom and passive wide field-of-view cameras, deployed in an upper floor of an office tower in downtown Toronto.
View more - IEEE Journal on Emerging and Selected Topics in Circuits and Systems
Journal of Electronic Imaging
Published in Automatic detection of road boundaries in traffic surveillance imagery can greatly aid subsequent traffic analysis tasks, such as vehicle flow, erratic driving, and stranded vehicles. This paper develops an online technique for identifying the dominant road boundary in video sequences captured by traffic cameras under challenging environmental and lighting conditions, e.g., unlit highways captured at night. The proposed method works in real time of up to 20 frames/s and generates a ranked list of road regions that identify road and lane boundaries. Results show that this method outperforms two state-of-the-art techniques in precision, recall, and runtime.Volume 24, Issue 5
Automatic detection of road boundaries in traffic surveillance imagery can greatly aid subsequent traffic analysis tasks, such as vehicle flow, erratic driving, and stranded vehicles. This paper develops an online technique for identifying the dominant road boundary in video sequences captured by traffic cameras under challenging environmental and lighting conditions, e.g., unlit highways captured at night. The proposed method works in real time of up to 20 frames/s and generates a ranked list of road regions that identify road and lane boundaries. Results show that this method outperforms two state-of-the-art techniques in precision, recall, and runtime.
CRV 2017 Computer Vision Best Paper
Computer Robot Vision (CRV 17) May 19, 2017CRV 2017 Computer Vision Best Paper for “Fast estimation of large displacement optical flow using dominant motion patterns & sub-volume patchmatch filtering,” selected by the awards committee as the best computer vision paper of the 14th Conference on Computer and Robot Vision (CRV 17), Edmonton, May, 2017.
ICDSC 2007 Outstanding Paper
IEEE September 28, 2007ICDSC 2007 Outstanding Paper for “Virtual Vision and Smart Cameras,” selected by the program committee as one of the best papers of the First ACM/IEEE International Conference on Distributed Smart Cameras, Vienna, Austria, September 2007. A refereed journal-length version was published in the Proceedings of the IEEE, 2008, Special Issue on “Distributed Smart Cameras.”
VSSN 2005 Outstanding Paper
Video Surveillance and Sensor Net-works (VSSN 05) November 11, 2005VSSN 2005 Outstanding Paper for article “Surveillance Camera Scheduling: A Virtual Vision Approach,” selected by the program committee as one of the best papers of the Third ACM International Workshop on Video Surveillance and Sensor Net- works (VSSN 05), Singapore, November 2005. An extended version was published in the ACM SIGMM journal Multimedia Systems, 2006, Special Issue on “Multimedia Surveillance Systems.”
Established and director of Visual Computing (VC) Lab
Dr. Qureshi established Ontario Tech University's state-of-the-art VC Lab which focuses on research problems that reside at the intersection of computer vision, visual sensor networks, and computer graphics.
Co-Chair Conference on Computer and Robot Vision
2016
Co-Chair of the 12th Conference on Computer and Robot Vision
June 1, 2015Co-Chair of the 12th Conference on Computer and Robot Vision in Halifax, Nova Scotia in June 2015, Dr. Qureshi will also Co-Chair next year's conference in Victoria British Columbia in June 2016.
Co-Chair 3rd IEEE Workshop on Camera Networks and Wide Area Scene Analysis
IEEECo-located with CVPR 2013
Co-Chair 2nd IEEE Workshop on Camera Networks and Wide Area Scene Analysis
IEEECo-located with CVPR 2012
Co-Chair IEEE Workshop on Camera Networks and Wide Area Scene Analysis
IEEECo-located with CVPR 2011